Computer Vision - HDR Network
High Dynamic Range, Why
Computer Vision - UNet from Autoencoder and FCN
UNet 可視為 Autoencoder 的一種變形,因為它的模型結構類似U型而得名。或是視為 FCN 的對稱改良版。
UNet 的 key ingredient: (1) 對稱的 encoder + decoder (from autoencoder); (2) bottleneck layer (from FCN); (3) (long) skip link from encoder to decoder (from FCN).
Improvement from UNet: (1) Add mixed long and short skip links; (2) Add more down-up sampling network
UNet 前世今生一:Autoencoder
Autoencoder 包含 encoder and decoder. Encoder 常常用來作爲 feature extract at low dimenstion. Decoder 剛好相反是升維,不過需要搭配 variational 手法 in training, i.e. variational autoencoder, 用於 image generation (e.g. MNIST number generation)
完整的 autoencoder, encoder+decoder, 是否有其他用途?答案是肯定的,可以用於 pixel-level image or video processing, 例如 segmentation, denoise, deblur, dehaze, etc.
Autoencoder 的主要問題是 quality. 在 encoder 降維做 feature extraction 的過程中,會丟失一些細節,只留下比較 high level information (e.g. object level feature)。之後的 decoder 從低維 upsampling 到高維的 image,可以視爲一種 filtering, 因爲缺乏細節,有可能會讓 output image 變得模糊。
很自然的想法就是提供高維的 skip link, 不至於讓高維的 information 在 decoder 降維都丟失。這就是 U-Net 的精神。
UNet 前世今生二:Fully Convolutional Network (FCN)
注意這裏 FCN 不是 fully connected network, 而是 fully convolutional network.
What and Why FCN?
对于一般的分类CNN网络,如VGG和Resnet,都会在网络的最后加入一些 (不止一層) 全连接层 (fully-connected layer, 如下圖 Hidden Layer H),经过softmax后就可以获得 class probability.
但是这个 probability information 是 1D 的,即只能标识整个图片的类别,不能标识每个像素点的类别。如下圖的 (a) 的 1D 4096/4096/1000 fully-connected layers. 所以这种全连接方法不适用于图像分割
而 FCN 提出可以把后面几个全连接 (fully connected layers) 都换成卷积 (convolutionalization), i.e. fully-connnected 1D 4096/4096/1000 layers 換成 convolutioned 2D 4095/4096/1000 layers. [longFullyConvolutional2015] 这样就可以获得一张 2D 的 feature map,后接softmax获得每个像素点的分类信息 (2D heat map),从而解决了分割问题,如下图 (b).
上面的缺點也很明顯,就是 2D heat map 的細緻度 (granularity) 不足。解決的方法就是用 up-sampling 得到 pixel-level 2D heat map 如下圖。
FCN Up-Sampling
FCN 使用的 up-sampling 如下圖。FCN paper 試了幾種 upsampling 的方法:
- 直接把 pool5 (down-sampling by 32) up-sampling x32, 稱爲 FCN-32s;
- Pool5 先 up-sampling x2, 再和 pool4 相加,再 up-sampling x16, 稱爲 FCN-16s;
- 把前面相加的結果再 up-sampling x2, 再和 pool3 相加,再 up-sampling x8, 稱爲 FCN-8s.
結果大概可以猜出來, FCN-8s > FCN-16s > FCN-32s, 如下圖。FCN-8s 最重要的 innovation 就是 shortcut from encoder to decoder, 這是之後 UNet 的濫殤。不確定 FCN 爲什麽不繼續做 FCN-4s or FCN-2s or FCN-s, 是結果沒有顯著的改善,還是其他的原因。
UNet 引入 shortcut 的觀念,同時又有點不同:
- Up-sampling 和 short-cut 的結合 在UNet 是用 concate,在 FCN 卻是相加。
- UNet encoder 和 decoder 是對稱的,每一層都有 shortcut from encoder to decoder.
- 是不是因爲 UNet 使用 concate 才能 enable all layer shortcut? TBC.
最後還有一個 trick, 有兩種 up-sampling 方法
-
Resize: use interpolation (e.g. bilinear or bicubic or bi???), 見另文。可視為 deconvolution 的 special case (fixed coefficient filter)
-
Deconvolution or Transposed Convolution
UNet 結構
UNet 可視為 Autoencoder 的一種變形,因為它的模型結構類似U型而得名。或是視為 FCN 的對稱改良版。如下圖。
U-Net 架構分分爲三個部分:(1) contraction (降維,類似 encoder);(2) bottleneck (from FCN, 見下文); (3) expansion (升維,類似 decoder):
-
Contraction: many blocks, each block 包含兩個 3x3 convolution layers followed by a 2x2 max pooling. The number of kernels or feature maps after layer dimension reduction 加倍。 目的是學習 high level features. Contraction 部分和一般 CNN network 非常類似或是一樣, e.g. VGG, or auto-encoder 的 encoder.
-
Expansion: 這是 U-Net 的核心部分。
- 基本的結構就是 reverse contraction 部分:each block 包含兩個 3x3 convolution layers followd by a 2x2 up-sampling layer. Feature maps or kernels after layer dimension expansion 減半。
- 重點:每個 block input 有兩個 inputs, 一個來自 up-sampling layer, 另一個來自對應的 encoder feature map, 兩者 equal size tensor concatenate. 這樣可以保證在 reconstruct output image 學到 encoder low level and high level feature maps. 我們把這種橫向的 connection 稱爲 short-cut.
-
Add short-cut from encoder to decoder. 這非常重要!如果沒有 short-cut 基本就是 autoencoder.
-
在變形的 U-Net, short-cut 並不限制在同一個 level from encoder-to-decoder. 可以有 encoder-to-encoder, 或是 decoder-to-decoder 長的或是短的 short-cut.
-
U-Net 基本變成影像處理網絡的 backbone and baseline, e.g. image segmentation, denoise, deblur, super resolution, HDR (high dynamic range), etc.
-
完整的 UNet 的參數有 xxx M 個,算力需求是 xx GOPs.
U-Net Rationale
基於 CNN 的網絡背後的主要想法是學習 feature map of an image, i.e. encoder. 這對image 分類問題效果很好,因為 image 先被轉換為 (one-dimension) vector,再進一步用於分類。 [sankesaraUNet2019]
但是在image 分割中,我們不僅需要將 feature map 轉換為 1D vector ,還需要從該 vector 重建 image, i.e. decoder. 這是一項艱鉅的任務,因為將 vector 轉換為 image (decoder) 比將 image 轉換為 vector (encoder) 要困難得多。 UNet 的整個想法就是圍繞這個問題展開的。
在將 image 轉換為 vector (encoder) 時,我們已經學習了 image 的 feature map,所以為什麼不使用相同的 mapping 將其再次轉換為 image。這是 UNet 背後的秘訣。
Use the same feature maps that are used for contraction to expand a vector to a segmented image. 這將保持 image 的結構完整性,從而極大地減少失真。
簡單說就是把 encoder 降維過程的 feature maps (包含 low level 的 feature) forward 到 decoder 融合到升維過程。
這是 pixel-level image task (e.g. segmentation, super resolution) 和 object-level image task (e.g. classification, detection) 本質上的不同。
UNet 結構改良
Resnet - Add Short skip Link
「从UNet的网络结构我们会发现两个最主要的特点,一个是它的U型结构,一个是它的跳层连接。」 其中UNet的编码器一共有4次下采样来获取高级语义信息,解码器自然对应了4次上采样来进行分辨率恢复,为了减少下采样过程带来的空间信息损失跳层连接被引入了,通过Concat的方式使得上采样恢复的特征图中包含更多low-level的语义信息,使得结果的精细程度更好。
使用转置卷积的UNet参数量是31M左右,如果对其channel进行缩小例如缩小两倍,参数量可以变为7.75M左右,缩小4倍变成2M左右,可以说是非常的轻量级了。UNet不仅仅在医学分割中被大量应用,也在工业界发挥了很大的作用。
我们知道UNet做下采样的BackNone是普通的CBR模块(Conv+BN+ReLU)堆叠的,一个自然的想法就是如果将学习更强的ResNet当作UNet的BackBone效果是否会更好呢? CVPR 2017的LinkNet给出了答案。LinkNet的网络结构如下所示:
Encoder block (with residue link) and decoder block
其中,conv
代表卷积,full-conv
代表全卷积 (fully convolutional?),/2
代表 down sampling 的 stride 是2
,*2
代表 up sampling 的因子是2
。 在卷积层之后添加 BN,后加 ReLU。左半部分表示编码,右半部分表示解码。编码块基于ResNet18
。
这项工作的主要贡献是在原始的UNet 的 encoder 引入了 residue or short skip link,并直接将编码器与解码器连接 (short-cut or long skip link) 来提高准确率,其實這也可以視爲是一種 residue link? 一定程度上减少了处理时间。通过这种方式,保留编码部分中不同层丢失的信息,同时,在进行重新学习丢失的信息时并未增加额外的参数与操作。在Citycapes 和 CamVID 数据集上的实验结果证明残差连接的引入(LinkNet without skip
)使得mIOU获得了提升。
这篇论文的主要提升技巧在于它的 skip 技巧,但我们也可以看到ResNet也进一步对网络的效果带来了改善,所以至少说明ResNet是可以当成BackBone应用在UNet的,这样结果至少不会差。
Local shortcut: D-LinkNet
CVPR 2018北邮在DeepGlobe Road Extraction Challenge全球卫星图像道路提取)比赛中勇夺冠军,他们提出了一个新网络名为D-LinkNet,论文链接以及代码/PPT见附录。
D-LinkNet使用LinkNet作为基本骨架,使用在ImageNet数据集上与训练好的ResNet作为网络的encoder,并在中心部分添加带有shortcut的dilated-convolution层,使得整个网络识别能力更强、接收域更大、融合多尺度信息。网络中心部分展开示意图如下:
这篇论文和ResNet的关系实际上和LinkNet表达出的意思一致,就是大量增加 short or long skip links 並将其应用在BackBone部分增强特征表达能力。
Mixed Long and Short Skip Links
这篇文章其实是比上两篇文章早的,但我想放到最后这个位置来谈一下,这篇文章是DLMIA 2016的文章,名为:「The Importance of Skip Connections in Biomedical Image Segmentation」 。这一网络结构如下图所示,对图的解释来自akkaze-郑安坤的文章
(a) 整个网络结构
使用下采样(蓝色):这是一条收缩路径。
上采样(黄色):这是一条不断扩大的路径。
这是一个类似于U-Net的FCN架构。
并且从收缩路径到扩展路径之间存在很长的跳过连接。
(b)瓶颈区
使用,因此称为瓶颈。 它已在ResNet中使用。
在每次转化前都使用,这是激活前ResNet的想法。
(c)基本块
两个卷积,它也用在ResNet中。
(d)简单块
个
卷积
(b)-(d)
所有块均包含短跳转连接。
下面的Table1表示整个网络的维度变化:
接下来是这节要分析的重点了,也就是长短跳过网络中两种不同类型的跳跃连接究竟对UNet的结果参生了什么影响?
这里训练集以张电子显微镜(EM)图像为数据集,尺寸为
。
张图像用于训练,其余
张图像用于验证。而测试集是另外
张图像。
下面的Figure3为我们展示了长短跳过连接,以及只有长跳过连接,只有短跳过连接对准确率和损失带来的影响:
(a)长跳和短跳连接
当长跳转和短跳转连接都存在时,参数更新看起来分布良好。
(b)仅长跳连接具有9个重复的简单块
删除短跳过连接后,网络的较深部分几乎没有更新。
当保留长跳连接时,至少可以更新模型的浅层部分。
(c)仅长跳连接具有3个重复的简单块
当模型足够浅时,所有层都可以很好地更新。
(d)仅长跳连接具有7个重复的简单块,没有BN。
论文给出的结论如下:
- 没有批量归一化的网络向网络中心部分参数更新会不断减少。
- 根据权值分析的结论,由于梯度消失的问题(只有短跳连接可以缓解),无法更有效地更新靠近模型中心的层。
所以这一节介绍的是将ResNet和UNet结合之后对跳跃连接的位置做文章,通过这种长跳短跳连接可以使得网络获得更好的性能。
B. UNet++ Add Middle Down-Up Sampling Network!
In addition to adding more links (short or long skip links), some ideas is to add down sampling convolution and up sampling network.
It’s very expensive to add those blocks. We only put it as a reference.
Pixel-Level Image Task
Pixel-level image task 的 training/inference/performance metrics 和 classifcation or detection 都有所不同。
此處我們討論 supervised learning, i.e. with labelled dataset.
Loss function 一般不是 cross-entropy loss (used in classification),而是 energy function.
Metrics 一般不是 accuracy, 而是 task dependent. 例如 segmentation 一般使用 IOU (Intersection Over Union); denoise 和 super resolution 一般用 PSNR (Peak Signal-to-Noise Ratio) 加上主觀評測。
以下我們用 image segmentation 爲例 illustrate U-Net 的 training for pixel-level image task.
Image Segmentation
下圖圖示 image segmentation 的作用。注意 image segmentation 并不需要分類。
Image segmentation 分爲 semantic segmentation 和 instance segmentation, 差異如下圖:
顯然 instance segmenation 比 semantic segementation 更困難。本文的 image segmentation 是 semantic segmentation.
Image Segmentation Performance Metrics (IoU, mIoU)
最常見的 performance metric 就是 IoU (Intersection-Over-Union) and Dice, 兩者的差異由下列公式和圖示看出: \(\operatorname{IoU}(A, B)=\frac{\|A \cap B\|}{\|A \cup B\|}, \quad \operatorname{Dice}(A, B)=\frac{2\|A \cap B\|}{\|A\|+\|B\|}\)
\[\text { IoU }=\frac{T P}{T P+F P+F N}, \quad \text { Dice }=\frac{2\, T P}{2\, T P+F P+F N}\] \[\text { IoU }=\frac{Dice}{2 - Dice}, \quad \text { Dice }=\frac{2 \, IoU}{1 + IoU}\]下圖紅色的正方形是 ground truth. 藍色的正方形是 predicted outcome.
IoU (or Dice) 和一般的 precision = $\frac{TP}{TP+FP}$ and recall = $\frac{TP}{TP+FN}$的定義稍有不同。
Both IoU and Dice 介於 (含) 0 and 1. 並且 IoU $\le$ Dice. 等號只有在 IoU or Dice = 1 (和 ground truth 完全重合) 或 0 (和 ground truth 完全不沾邊) 成立。下面的例子也可以看出 IoU 和 Dice 的大小關係。一般我們用 IoU.
IoU 是針對一個 class (e.g. person) 的結果。mIoU (mean IoU) 是對所有 classes (e.g. sky, sidewalk, etc.) 的平均結果。
Loss Function
The energy function is computed by a pixel-wise soft-max over the final feature map combined with the cross-entropy loss function
UNet uses a rather novel loss weighting scheme for each pixel such that there is a higher weight at the border of segmented objects. This loss weighting scheme helped the U-Net model segment cells in biomedical images in a discontinuous fashion such that individual cells may be easily identified within the binary segmentation map.
First of all pixel-wise softmax applied on the resultant image which is followed by cross-entropy loss function. So we are classifying each pixel into one of the classes. The idea is that even in segmentation every pixel have to lie in some category and we just need to make sure that they do. So we just converted a segmentation problem into a multiclass classification one and it performed very well as compared to the traditional loss functions.
聽起來是 two-tier loss function: tier1 是 all pixel-wise softmax (using cross-entropy loss?); tier 2 是 weighting for different pixel, 特別是 boundary 的 weighting 比較重。不過我 trace code, 只有看到 cross-entropy loss?
實際的 PyTorch code [sankesaraUNet2019] 可參考 reference A
Appendix
Appendix A: PyTorch Code Review (Kaggle Segmentation of OCT Image, DME)
UNet 的結構 (forward) 包含 Encoder, Bottleneck, Decode 三個部分
Encode 非常簡單:就是 3 個 (contracting block + MaxPool2D), (比上圖的結構少一層)
Contracting block:(Conv2d+ReLU+BatchNorm) + (Conv2d+ReLU+BatchNorm)
好像不需要把 MaxPool2D 放在 contracting block 外面?
Bottleneck block 次簡單:最後一層 up-sample by 2
(Conv2d+ReLU+BatchNorm) + (Conv2d+ReLU+BatchNorm) + ConvTranspose2d : (Stride=2 for up-sampling). => 其實和 expansive block 相同,只是沒有 crop-and-concat.
Decode 比較麻煩:多了 short-cut, 也是 3 個 (cat + expansive block)
Expansive block : (Conv2d+ReLU+BatchNorm) + (Conv2d+ReLU+BatchNorm) + ConvTranspose2d : (Stride=2 for up-sampling x2)
最後一個 expansive block 稱爲 final block. 因爲不需要再 up-sampling, 把 ConvTranspose2d 改回 Conv2d+ReLU+BatchNorm
1 |
|
其他部分就是 loss (cross-entropy) 和 optimizer (SGD)
1 |
|
Use the example to compare U-Net vs. Auto-encoder by removing the short-cut!
Autoencoder 用於 image processing 第一個問題是如何 training.
- dataset (label?)
- loss function
- supervise or self-supervised
SR,
HDR
NR,
再來我們看比較複雜的影像處理 HDR (High Dynamic Range), 代表的網路是 HDRNet
HDR
Computer Vision - FRC and MEMC
Main Reference
- [@santamariaEntropyMutual2015]
- [@baoMEMCNetMotionEstimation2019]
- [@parkBMBCBilateral2020]
FRC - Frame Rate Conversion
FRC 顧名思義就是做幀率轉換,一般是從低幀率轉換成高幀率。爲什麽需要做 FRC? 有兩個原因:(1) bridge video/game content frame rate and device refresh rate; (2) 高幀率 (HFR - High Frame Rate) 提高 video or game 的流暢性 (smoothness),這是目前主流或是高階電視和手機的賣點。
Video Content Frame Rate
大多電影拍攝還是 native 24 FPS (李安的 Billy Lynn’s Long Halftime Walk 是史上最高電影使用 native 120 FPS). TV 和 Game 大多是 native 30, 60 (少數 120 FPS). Video call 例如 WeChat, Line, Zoom 可能用不到 10 FPS 的 video stream 節省頻寬和遲延 (latency).
Device Refresh Rate
主流電視 (2021) 的 refresh rate 是 60Hz; 高階電視可以到 120Hz, 甚至 240Hz (大多是插黑幀)。主流旗艦手機 (2021) 的 refresh rate 是 120Hz.
下表 summarize video content 和 device refresh rate 的 gap, 需要 FRC to bridge the gap.
Video Cotent Frame Rate | Device Refresh Rate |
---|---|
Movie: 24 FPS | TV device: 60/120/240Hz |
TV video: 30, 60 FPS | Smart phone device: 60/90/120Hz |
Game: 30, 60, 90 FPS | |
Video call: 15/10 FPS |
Smoothness
以上是單從 bridge video/game content and device refresh rate 出發。如果只是這個目的,最簡單的方法就是插重複幀,或者插黑幀。
我們舉一個實際的例子,例如 movie content 是 24 FPS, 但要在 60 Hz TV 顯示。 最簡單的方法就是如下的插幀。
不單是插重複幀,而且還不是均匀插幀。而是 2-3-2-3 插幀。兩個問題 (1) 不均匀插幀會造成視覺上有顫抖 (judder) 現象,Youtube 有一些影片可以參考;(2) 低 frame rate (e.g. 24 FPS) 雖然有所謂的 “電影感”, 但在内容有高速運動的畫面 (e.g. 球賽或是打鬥) 就會顯得模糊1。
實務上沒有任何電視會用這種方法做 24 to 60 FPS 的 FRC. 這就會帶到下一個題目, MEMC.
MEMC - Motion Estimation Motion Compensation
FRC 是一個規格需求,從 A FPS 轉到 B FPS, 一般 B > A. 最簡單的做法是(不均匀)重複插幀或是插黒幀。不過沒有電視這樣做,因爲視覺感受 (visual perception) 不好。
目前主流的方法就是對 native frames, e.g. N and N+1 at 24 FPS, 做 motion estimatin (ME), 再根據要插出的幀的時間做 motion compensation (MC), e.g. M, M+1, …, M+5 at 60 FPS, 也就是 2 個 input frames 產生 5 個 output frames (24FPS to 60FPS). ME 加 MC 合稱爲 MEMC.
Forward and Backward Motion Map
我們用一個最簡單也是目前最常用的 MEMC 爲例,如下圖: input frame rate N at 30FPS, output frame rate M at 60 FPS, conversion rate 為 2. 兩張 input frames, 叫做 $I_0$ and $I_1$, 可以計算或搜尋出 forward motion (vector) map, 就是 image 的每一個 pixel 都有對應的 motion vector, 稱爲 $F_{0\to1}$. 這個過程就是 ME (motion estimation). 再來藉助 $I_0$ 和 $F_{0\to 1}$,就可以 forward warp 出 $I_{0.5}$ output frame. 這個過程就是 MC (motion compensation).
In summary: ME 就是產生 motion map; MC 就是用原來的 image 加上 motion map, warp 出 output image.
上述 forward warp 並不是唯一的 ME-MC。我們也可以計算 backward motion (vector) map, 稱爲 $F_{1\to 0}$, 藉助 $I_1$ 和 $F_{1\to 0}$, 同樣可以 backward warp 出 $I_{0.5}$ output frame.
乍看之下,好像 forward motion map $F_{0\to 1}$ 和 backward motion map $F_{1\to 0}$ 是 reverse 的關係。其實不然。
以下圖 original image 爲例,$F_{0\to 1}$ 在 $I_0$ 小車的位置的 motion vector 是前進,其他位置為 0. 但 $F_{1\to 0}$ 雖然 motion vector 是後退,和 $F_{0\to 1}$ 剛好是 reverse,但卻是在 $I_1$ 的小車位置,而不是在 $I_0$ 小車的位置,一般我們稱爲不同的 anchor point (錨定點)。所以 forward motion map $F_{1\to 0}$ 不是 backward motion map $F_{0\to 1}$ 的 reverse map,因爲 moving object 在不同 frame 的 anchor point 不同!
More problem when occulusion occurs
The motion map is NOT 1-to-1, but many-to-1!!
Back to Physics (Laplace Monster!)
current object position and velocity, we can predict everything
Problem, we don’t have velocity => easy, we use t_0 and t_1 for the velocity
Problem, 2D and 3D
Problem, new information, for example, a monkey jumping from the stone at t1 (I don’t know where it is in t_0.5)! or move to a new scene! => inpainting, best guess
Video use both forward and backward and blending for more information!
爲了讓 picture quality 更好,一般我們也會估算 backward motion vector, 稱爲 $F_{1\to 0}$
MEMC 的技術基本分爲 (1) 傳統的方法和 (2) 深度學習的方法 [wikiVideoSuperresolution2021].
此處略過 (1),主要介紹 (2) 深度學習的 MEMC.
Challenge
- 3D to 2D so information is not complete, occusion problem without depth map!! (ill-condition)
- 2D video Interpolation will suffer from the occusion problem
- 3D graphics (with depth map) interpolation does not have this problem, but graphic will not use this because of latency
- Extrapolation has additional problem of information deficiency (ill-condition)
- 2D Video will not use it because it’s quality loss, and it can tolerate latency
- 3D Graphic suffer from this problem
MEMC Basic And Challenges
顧名思義,MEMC 分爲兩個部分: ME (Motion Estimation) and MC (Motion Compensation).
Deep Learning Based MEMC
Deep Learning Based 可以分爲兩類:(A) ME and MC; (B) Deformable convolution.
ME-MC
Motion Estimation: provide information about the motion of pixels between frames.
Motion Compensation: a warping operation, which aligns one frame to another based on motion information.
ME / MC | Two frames Interpolation | One Frame Extrapolation |
---|---|---|
one motion | ||
Game, Motion/depth map from ground truth | forward warping | |
XR, Motion/depth map from IMU sensor | forward warping | |
Video, Motion/visual maps from consecutive frames | Prefer backward warping for better quality: ideal two images blending, no inpainting; but has image halo issueto solve motion map halo (use motion map inpainting) | Forward warping problem: 1. predicted motion map error cause overshoot; 2. object occulusion w/o depth map; 3 Image inpainting |
對於 video 而言,ME 主要由連續 frames 產生,例如 optical flow. 基於 optical flow 的 深度學習有 FlowNet, RAFT, etc. 可以參考上文。
對於 game 而言,ME 有機會事先得知 (object motion based on physics), 不一定要用最後的 image frames 產生。
Aligned by deformable convolution
一般 image 的 convolution 都是 fixed kernel (e.g. CNN). Deformable convolution 則是先 estimate shifts for kernel and do convolution.
3D Convolution
motion estimation: optical flow
AI-MEMC
AI motion estimation
FlowNet
RAFT
Kernel method
AI motion compensta
MEMC
- CV
- AI optical flow - pixel level
- Kernel - patch level
Motion Estimation Motion Compensation (MEMC)
Optical flow 的一個主要應用是 MEMC,就是所謂插幀。基本所有的電視都有這個功能。就是從 $I_{t-1}$ 和 $I_{t+1}$ 内插出 $I_t$. 這個插幀可以在正中間,例如 30 FPS to 60 FPS; 或是 60 FPS to 120 FPS. 也可以不在正中間,例如 24 FPS to 60 FPS.
MEMC 顧名思義包含 ME (Motion Estimation) 和 MC (Motion Compensation).
ME 基本有三類: (i) conventional ME (此處不論); (ii) optical flow motion estimation (pixl level); (iii) kernel (patch level) (也不論)。
MC 包含: image warping,image inpainting.
因爲深度學習的 optical flow motion estimation 已經包含 image/feature warping, 因此 ME 和 MC 可以合在同一個網路。就是把原來 optical flow network for motion estimation 擴大 to cover 完整的 MEMC network。
基本可以把 MEMC 分爲三個 steps,如下圖: [@baoMEMCNetMotionEstimation2019]
Step 1: 我們可以從 $I_{t-1}$ 和 $I_{t+1}$ 得到 forward optical flow $f_{t-1\to t+1}$, 和 backward optical flow $f_{t+1\to t-1}$.
Step 2: 再來是從 step 1 的 optical flow 内插 $f_{t\to t-1}$ 和 $f_{t\to t+1}$
Step 3: 接下來觀念上可以用 $I_{t-1} + f_{t\to t-1}$ backward warping 得到 $I_t$. 同樣用 $I_{t+1} + f_{t\to t+1}$ backward warping 得到 $I_t$. 當然這兩個結果還是會有差異。因此觀念上可以做 bilaterial warping 得到更好的結果。
接下來會看一些例子。
Ex1: MEMC-Net (2019),ME is based on FlowNetS
[@baoMEMCNetMotionEstimation2019] 下圖是 MEMC-Net architecture. 最上面的分支就是 Motion Estimation.
ME part
Step 1: Motion estimation 直接用 FlowNetS in Fig. 3. Input: $I_{t-1}, I_{t+1}$, output: $f_{t-1\to t+1}, f_{t+1\to t-1}$.
Step 2: 用 flow projection layer, input: $f_{t-1\to t+1}, f_{t+1\to t-1}$, output $f_{t\to t+1}, f_{t\to t-1}$. 基本假設 linear motion projection.
MC part
Warping : motion warping + kernel warping
Inpainting: 因爲有兩張 frames, 一般會有 1 frame occlusion 可以被另一 frame cover. 所以只要標出 Occulusion mask 配合 warping 即可。最後如 PWCnet 再加上 context network for post processing.
再看一個例子,BMBC:
Ex2: BMBC (Bilateral Motion Estimation with Bilateral Cost Volume, 2020), based on PWCNet
[@parkBMBCBilateral2020] 下圖是 BMBC archtecture. 上三路的 (shared) bilateral motion network 是最重要的 building block to perform Motion Estimation (ME)。之後的 warping layer 和第四路的 context extractor 則是 perform Motion Compensation (MC)。
ME part
Combine Step 1 and 2: Bilateral Motion Estimation,如下圖。這裏把 step 1 和 2 結合一起,直接得到 $V_{t\to 0}$ 和 $V_{t\to 1}$,如下圖。
其實是把 PWCnet 加上改良,把原來 Pyramid1 warp to Pyramid0 部分,再加上 Pyramid0 warp to Pyramid1 (改良 bilateral 部分)。比較巧妙的部分是直接把兩個改成 Pyramid1/2 warp to Pyramid t. 並且得到 $V^l_{t\to 0}$ and $V^l_{t\to 1}$。 注意這裏都是用 backward warping!
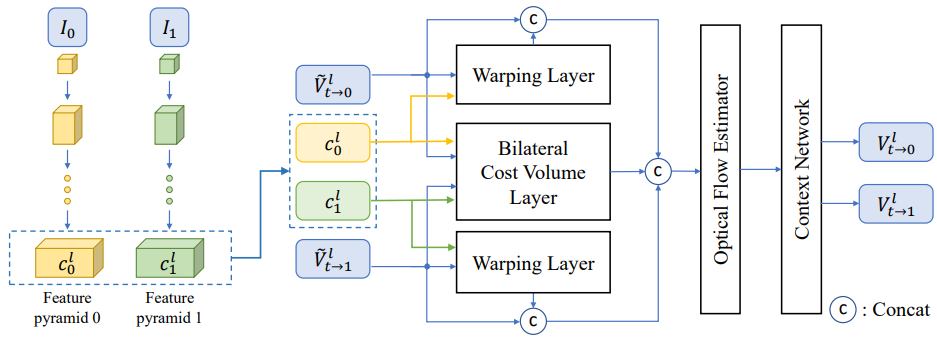
Cost Volume 的做法也是變成雙向。$d$ 是 search window size $D = [-d, d] \times [-d, d]$ 爲了減小 computation complexity.
\(B C_{t}^{l}(\mathbf{x}, \mathbf{d})=c_{0}^{l}\left(\mathbf{x}+\widetilde{V}_{\mathrm{t} \rightarrow 0}^{l}(\mathbf{x})-2 t \times \mathbf{d}\right)^{T} c_{1}^{l}\left(\mathbf{x}+\widetilde{V}_{\mathrm{t} \rightarrow 1}^{l}(\mathbf{x})+2(1-t) \times \mathbf{d}\right)\)
注意 $V_{0\to 1}$ 或是 $V_{1\to 0}$ 只是 $t=0$ 或是 $t=1$ 的特例。就回到 PWC-Net.
那麽上上圖的 branch 1 and 3 的 Motion Approximation 是要做什麽? 主要是針對 occlusion 再產生更多的 $V_{t\to 0}$ 和 $V_{t\to 1}$,如下圖。細節請直接參考 paper.
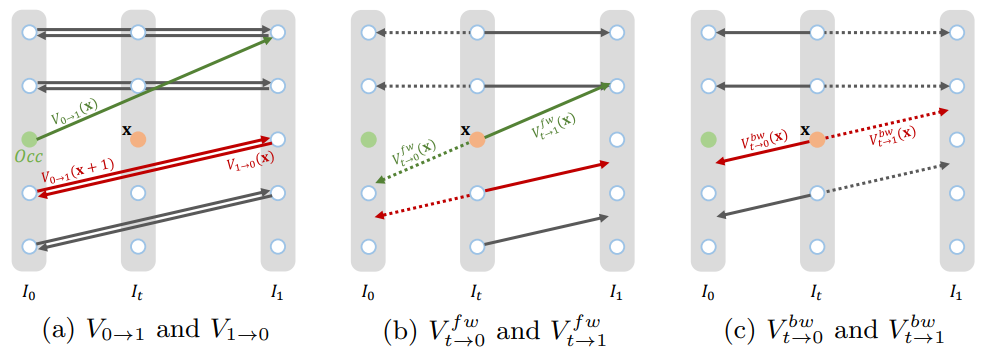
再來非常複雜的把三路中每一路的 4 張 estimated Image t, 連同 2 張 input image, 一共 4x3+2 = 14 張合成 $I_t$. 應該是不計計算成本。
實驗結果
用了 4 組 datasets, Middlebury, Vimeo90K, UCF101, Adobe240-fps. 並且比較 SOTA 結果。
- Adaptive convolution: SepConv, ToFlow, CtxSyn
- Optical Flow NN: ToFlow, SPyNet, MEMC-Net (Bao), DAIN (depth aware, Bao), BMBC
Middlebury
-
電影一般用特效 (e.g. slow motion) 處理。 ↩
Excel Link to MySQL
作為同門 Microsoft 的資料庫產品,Excel操作Access、SQLServer中的數據非常簡單。但如果想在Excel中處理MySQL中的數據呢?MySQL是和 php 珠聯璧合的一個資料庫,網站開發中經常用到,如果Excel也能訪問它就會給工作帶來很多方便。
最直接的應用就是把現有的 excel table 匯出到 MySQL table 避免重新鍵入資料。
另外的應用是 excel 讀出 MySQL table. 例如,MySQL資料庫中的用戶登錄數據表,有時希望在Excel中對該表進行一些分析:
原文網址:
https://kknews.cc/code/pqbjrv8.html
https://kknews.cc/code/pqbjrv8.html
https://www.youtube.com/watch?v=qK9gPEF606U&ab_channel=SyntaxByte (MySQL -> Excel, very good YouTube)
從 MySQL 匯出到 Excel (比較容易)
具體操作步驟:
Step 1: 到 MySQL website download ODBC connector driver and install
https://dev.mysql.com/downloads/connector/odbc/
重點是確認 32-bit or 64-bit version. 不過 Windows 10 之後 Excel 都是 64-bit version. 好像也不是什麽問題。
After installation, go to command window and search odbc
Then, add MySQL ODBC Unicode Driver
Then setup the MySQL connection and test it.
此時 ODBC 多了 MySQL local!
Step 2: 到 Excel build the MySQL database link
Now we go to Excel
Data tab -> Get Data -> from other sources -> from ODBC
Choose MySQL local
下一步是關鍵! Choose Default or Custom! and leave blank!
就會出現 MySQL 目前的所有 database in Navigator window
可以直接 navigate the database and load the table
或是 Transform the data if needed.
從 Excel 匯入到 MySQL (比較複雜)
- Local load csv file
- Use Python (or PHP) to insert csv file to MySQL
- Use Excel ODBC ? (no direct way?)
Method | Local or Remote | Format |
---|---|---|
Manual | Local load | csv |
Python (or PHP) | Remote | csv |
Commercial tool | Remote | excel |
Method 0: Use 3rd party tool
Ex1: Navicat https://www.gushiciku.cn/pl/gQIF/zh-tw => 非常貴!
Method 1: Local load csv file
https://chartio.com/resources/tutorials/excel-to-mysql/
-
Download the boats.xlsx file, open in excel, and save as (windows) csv file.
-
Log into the MySQL shell and create a database. For this example the database is named boatdb. Not that the –local-infile option is needed by some version of MySQL for the data loading.
-
$ mysql -u root -p –local-infile
-
mysql> create database boatdb;
-
mysql> use boatdb;
-
Then define the schema for our boat table using the CREATE TABLE
CREATE TABLE boats ( id INT NOT NULL PRIMARY KEY, name VARCHAR(40), type VARCHAR(10), owner_id INT NOT NULL, date_made DATE, rental_price FLOAT );
-
-
檢查是否 create database and table ok.
-
$ mysql> show tables;
-
-
再來就是最關鍵的部分,LOAD DATA command.
LOAD DATA LOCAL INFILE "c:/Users/allen/Downloads/boats.csv" INTO TABLE boatdb.boats
FIELDS TERMINATED BY ','
LINES TERMINATED BY '\n'
IGNORE 1 LINES
(id, name, type, owner_id, @datevar, rental_price)
set date_made = STR_TO_DATE(@datevar,'%m/%d/%Y');
結果失敗了!應該是 permission 的問題。
ERROR 2068 (HY000): LOAD DATA LOCAL INFILE file request rejected due to restrictions on access.
我費了九牛二虎之力都無法解決在 sql shell LOAD DATA LOCAL INFILE 的問題!
最後我找到 solution,就是直接在 workbench 的 command window 就可以!
接下來就可以做各種 query!
Method 2: Python or PHP
之前 MySQL 的管理 SW 最普遍的是 phpMyAdmin, 主要是用 PHP script 作爲 front-end interface (html) 和 backend database 溝通的工具。
之後 MySQL 提供的 workbench, 是由 Python 寫的。很自然也是用 Python 作爲和 database 溝通的工具。
以下是兩者的比較:
PHPMyAdmin (PHP)
Pros
- Commonly installed on managed hosting environments
- Web Based which means you can access from any computer
- Local resources aren’t used when connecting
- Simplicity
Cons
- No schema visualization
- If remote database working offline can be more difficult
MySQL Workbench (Python)
Pros
- Saved SQL statements
- Offline access to remote DB’s
- Handle/Store multiple connections in one location
Cons
- Resource consumption
- More complex than the average user would need
參考 [cheahHowUse2019] and [projectproHowConnect2020],此處我們用 Python connector access the database. 這裏還有一些設定上的插曲。Windows 的 Workbench install Python connector 不是很順利,最後是用 Windows anaconda create a virtual environment,再 install python connection. 嚴格來説和 workbench 沒有一毛錢的關係。
1 |
|
程式碼分爲三部分
- Read CSV file using pandas.
- Create a database.
- Create a table and insert csv records to the table.
1 |
|
使用 Python 還有一個潛在的好處,就是可以用來做 ML/AI 分析。此處不再贅述。
Reference
Math ML - Entropy and Mutual Information
HMM Triology (III) - EM Algorithm
Math AI - VAE Coding
Main Reference
-
[@kingmaIntroductionVariational2019] : excellent reference
-
[@kingmaAutoEncodingVariational2014]
-
[@roccaUnderstandingVariational2021]
VAE Recap
Recap VAE spirit: marginal likelihood = ELBO + gap => focus on ELBO only!
\[\begin{aligned}\log p_{\boldsymbol{\theta}}(\mathbf{x}) &=\underbrace{\mathbb{E}_{q_{\boldsymbol{\phi}}(\mathbf{z} \mid \mathbf{x})}\left[\log \left[\frac{p_{\boldsymbol{\theta}}(\mathbf{x}, \mathbf{z})}{q_{\boldsymbol{\phi}}(\mathbf{z} \mid \mathbf{x})}\right]\right]}_{=\mathcal{L}_{\theta,\phi}{(\boldsymbol{x}})\,\text{, ELBO}}+\underbrace{\mathbb{E}_{q_{\phi}(\mathbf{z} \mid \mathbf{x})}\left[\log \left[\frac{q_{\boldsymbol{\phi}}(\mathbf{z} \mid \mathbf{x})}{p_{\boldsymbol{\theta}}(\mathbf{z} \mid \mathbf{x})}\right]\right]}_{=D_{K L}\left(q_{\boldsymbol{\phi}}(\mathbf{z} \mid \mathbf{x}) \| p_{\boldsymbol{\theta}}(\mathbf{z} \mid \mathbf{x})\right)}\end{aligned}\] \[\begin{aligned}\underbrace{\mathbb{E}_{q_{\boldsymbol{\phi}}(\mathbf{z} \mid \mathbf{x})}\left[\log \left[\frac{p_{\boldsymbol{\theta}}(\mathbf{x}, \mathbf{z})}{q_{\boldsymbol{\phi}}(\mathbf{z} \mid \mathbf{x})}\right]\right]}_{=\mathcal{L}_{\theta,\phi}{(\boldsymbol{x}})\,\text{, ELBO}} &= \mathbb{E}_{q_{\phi}(\mathbf{z} | \mathbf{x})}\left[\log p_{\theta}(\mathbf{x} | \mathbf{z})\right] - D_{K L}\left(q_{\phi}(\mathbf{z} | \mathbf{x}) \|\,p(\mathbf{z})\right) \\&= (-1) \times \text{VAE Loss Function}\end{aligned}\]With the loss function, We can start training.
- Gradient
- Some term are samples (1), some has analytical form (2) (see appendix A)
(1) Naive Monte Carlo gradient estimator
$\nabla_{\phi} E_{q_{\phi}(\mathbf{z})}[f(\mathbf{z})] = E_{q_{\phi}(\mathbf{z})}\left[f(\mathbf{z}) \nabla_{q_{\phi}(\mathbf{z})} \log q_{\phi}(\mathbf{z})\right] \simeq \frac{1}{L} \sum_{l=1}^{L} f(\mathbf{z}) \nabla_{q_{\phi}\left(\mathbf{z}^{(l)}\right)} \log q_{\phi}\left(\mathbf{z}^{(l)}\right)$
where $\mathbf{z}^{(l)} \sim q_{\phi}\left(\mathbf{z} \mid \mathbf{x}^{(i)}\right)$.
This gradient estimator exhibits exhibits very high variance (see e.g. [BJP12])
SGVB estimator and AEVB algorithm
這節討論實際的 estimator of approximate posterior in the form of $q_\phi(\mathbf{z}\mid \mathbf{x})$. 注意也可以適用於 $q_\phi(\mathbf{z})$.
Under certain mild conditions outlined in section 2.4 for a chosen approximate posterior $q_\phi(\mathbf{z}\mid \mathbf{x})$ we can reparametrize the random variable $\tilde{\mathbf{z}} \sim q_\phi(\mathbf{z}\mid \mathbf{x})$ using a differentiable transformation $g_{\phi}(\epsilon, x)$ of an (auxiliary) noise variable :
\[E_{q_{\phi}\left(\mathbf{z} \mid \mathbf{x}^{(i)}\right)}[f(\mathbf{z})]=E_{p(\epsilon)}\left[f\left(g_{\phi}\left(\boldsymbol{\epsilon}, \mathbf{x}^{(i)}\right)\right)\right] \simeq \frac{1}{L} \sum_{l=1}^{L} f\left(g_{\phi}\left(\boldsymbol{\epsilon}^{(l)}, \mathbf{x}^{(i)}\right)\right) \quad$ where $\quad \boldsymbol{\epsilon}^{(l)} \sim p(\boldsymbol{\epsilon})\]We apply this technique to the variational lower bound (eq. (2)), yielding our generic Stochastic Gradient Variational Bayes (SGVB) estimator $\widetilde{\mathcal{L}}^{A}\left(\boldsymbol{\theta}, \boldsymbol{\phi} ; \mathbf{x}^{(i)}\right) \simeq \mathcal{L}\left(\boldsymbol{\theta}, \boldsymbol{\phi} ; \mathbf{x}^{(i)}\right)$ :
\[\widetilde{\mathcal{L}}^{A}\left(\boldsymbol{\theta}, \boldsymbol{\phi} ; \mathbf{x}^{(i)}\right)=\frac{1}{L} \sum_{l=1}^{L} \log p_{\boldsymbol{\theta}}\left(\mathbf{x}^{(i)}, \mathbf{z}^{(i, l)}\right)-\log q_{\phi}\left(\mathbf{z}^{(i, l)} \mid \mathbf{x}^{(i)}\right)\]where $\quad \mathbf{z}^{(i, l)}=g_{\phi}\left(\boldsymbol{\epsilon}^{(i, l)}, \mathbf{x}^{(i)}\right) \quad$ and $\quad \boldsymbol{\epsilon}^{(l)} \sim p(\boldsymbol{\epsilon})$
Algorithm 1: Minibatch version of Auto-Encoding Variational Bayes (AEVB) algorithm. We set M=100 and L=1
$\theta, \phi$ : Initialize parameters
Repeat
-
$X^M$ Random minibatch of M datapoints (drawn from full dataset)
-
$\boldsymbol{\epsilon}$ Random samples from noise distribution $p(\boldsymbol{\epsilon})$
-
$\mathbf{g}$ gradients of minibatch estimator
-
$\theta, \phi$ Update parameters using gradients $\mathbf{g}$
??? SGVB estimator $\widetilde{\mathcal{L}}^{B}\left(\boldsymbol{\theta}, \boldsymbol{\phi} ; \mathbf{x}^{(i)}\right) \simeq \mathcal{L}\left(\boldsymbol{\theta}, \boldsymbol{\phi} ; \mathbf{x}^{(i)}\right)$, corresponding to eq. (3), which typically has less variance than the generic estimator:
\[\widetilde{\mathcal{L}}^{B}\left(\boldsymbol{\theta}, \boldsymbol{\phi} ; \mathbf{x}^{(i)}\right)=-D_{K L}\left(q_{\boldsymbol{\phi}}\left(\mathbf{z} \mid \mathbf{x}^{(i)}\right) \| p_{\boldsymbol{\theta}}(\mathbf{z})\right)+\frac{1}{L} \sum_{l=1}^{L}\left(\log p_{\boldsymbol{\theta}}\left(\mathbf{x}^{(i)} \mid \mathbf{z}^{(i, l)}\right)\right)\]where $\quad \mathbf{z}^{(i, l)}=g_{\phi}\left(\boldsymbol{\epsilon}^{(i, l)}, \mathbf{x}^{(i)}\right) \quad$ and $\quad \boldsymbol{\epsilon}^{(l)} \sim p(\boldsymbol{\epsilon})$
Given multiple datapoints from the dataset $X$ with N datapoints, we can
\[\mathcal{L}(\boldsymbol{\theta}, \boldsymbol{\phi} ; \mathbf{X}) \simeq \widetilde{\mathcal{L}}^{M}\left(\boldsymbol{\theta}, \boldsymbol{\phi} ; \mathbf{X}^{M}\right)=\frac{N}{M} \sum_{i=1}^{M} \widetilde{\mathcal{L}}\left(\boldsymbol{\theta}, \boldsymbol{\phi} ; \mathbf{x}^{(i)}\right)\]Example: Variational Auto-Encoder, assuming Gaussian
\[\mathcal{L}\left(\boldsymbol{\theta}, \boldsymbol{\phi} ; \mathbf{x}^{(i)}\right) \simeq \frac{1}{2} \sum_{j=1}^{J}\left(1+\log \left(\left(\sigma_{j}^{(i)}\right)^{2}\right)-\left(\mu_{j}^{(i)}\right)^{2}-\left(\sigma_{j}^{(i)}\right)^{2}\right)+\frac{1}{L} \sum_{l=1}^{L} \log p_{\theta}\left(\mathbf{x}^{(i)} \mid \mathbf{z}^{(i, l)}\right)\]where $\quad \mathbf{z}^{(i, l)}=\boldsymbol{\mu}^{(i)}+\boldsymbol{\sigma}^{(i)} \odot \boldsymbol{\epsilon}^{(l)} \quad$ and $\quad \boldsymbol{\epsilon}^{(l)} \sim \mathcal{N}(0, \mathbf{I})$
VAE Encoder-Decoder Structure
From [@roccaUnderstandingVariational2021],一個是 encoder NN, 如下式 $(g^, h^)$
\[\begin{aligned} \left(g^{*}, h^{*}\right) &=\underset{(g, h) \in G \times H}{\arg \min } K L\left(q_{x}(z), p(z \mid x)\right) \\ &=\underset{(g, h) \in G \times H}{\arg \max }\left(\mathbb{E}_{z \sim q_{x}}\left(-\frac{\|x-f(z)\|^{2}}{2 c}\right)-D_{K L}\left(q_{x}(z), p(z)\right)\right) \end{aligned}\]另一個是 decoder NN, 如下式 $f^*$
\[\begin{aligned} f^{*} &=\underset{f \in F}{\arg \max } \mathbb{E}_{z \sim q_{x}^{*}}(\log p(x \mid z)) \\ &=\underset{f \in F}{\arg \max } \mathbb{E}_{z \sim q_{x}^{*}}\left(-\frac{\|x-f(z)\|^{2}}{2 c}\right) \end{aligned}\]Gathering all the pieces together, we are looking for optimal $\mathrm{f}^{}, \mathrm{~g}$ and $\mathrm{h}^{*}$ such that
\[\left(f^{*}, g^{*}, h^{*}\right)=\underset{(f, g, h) \in F \times G \times H}{\arg \max }\left(\mathbb{E}_{z \sim q_{x}}\left(-\frac{\|x-f(z)\|^{2}}{2 c}\right)-D_{K L}\left(q_{x}(z), p(z)\right)\right)\]等價於 minimize VAE loss function
\[\begin{aligned} \text {VAE loss }&=C\|x-\hat{x}\|^{2}+D_{KL}\left(N\left(\mu_{x}, \sigma_{x}\right), N(0, I)\right)\\ &=C\|x-f(z)\|^{2}+D_{KL}(N(g(x), h(x)), N(0, l)) \end{aligned}\]第一項是 reconstruction loss, 第二項是 regularization loss. 第一項從 sampling 得到。第二項有 analytical form, 見 Appendix A.
In practice, g and h are not defined by two completely independent NN but share a part of their architecutre and theier weights so that
$\mathbf{g}(x) = \mathbf{g}_2(\mathbf{g}_1(x)) \quad \mathbf{h}(x) = \mathbf{h}_2(\mathbf{h}_1(x)) \quad \mathbf{g}_1(x) = \mathbf{h}_1(x)$
Binary Image Approximation Using Bernoullie Distribution
如果 image 是黑白二值 (binary black and white), 可以用 Bernoulli distributionm. Reconstruction loss 可以改用 binary cross entropy loss, 而不是 上面的 MSE loss.1
\(p(\xi)=\left\{\begin{array}{l} \rho, \xi=1 \\ 1-\rho, \xi=0 \end{array}\right.\) Bernoulli distribution 適用於多個二值向量的情况,比如 $x$ 是 binary image (mnist可以看成這種例子,雖然是 grey value 而不是 binary value) \(q(x \mid z)=\prod_{k=1}^{D}\left(\rho_{(k)}(z)\right)^{x_{(k)}}\left(1-\rho_{(k)}(z)\right)^{1-x_{(k)}}\) \(-\ln q(x \mid z)=\sum_{k=1}^{D}\left[-x_{(k)} \ln \rho_{(k)}(z)-\left(1-x_{(k)}\right) \ln \left(1-\rho_{(k)}(z)\right)\right]\)
這表明 $\rho(z)$ 要把 output 壓縮在 0~1 (例如用 sigmoind activation), 然後用 BCE 做為 reconstruction loss function,
以下是 VAE PyTorch code example for MNIST
MNIST dataset
- MNIST image: size 28x28=784 pixels of grey value between 0 and 1. 0: 白;1:黑。0.1-0.9 代表不同的灰階,如下圖。
- MNIST datset: 60K for training; 10K for testing. Total 70K.
VAE Model
- VAE encoder first uses FC network (fc1: 784->400) + ReLU, 等價上圖的 $\mathbf{h}_1 = \mathbf{g}_1$
- 再接上兩個 FCs (fc21=$\mathbf{g}_2$, fc22=$\mathbf{h}_2$, 400->20) 產生 mean,mu, and log of variance, logvar of 20 dimensions. 注意這二個 FCs 沒有串接 ReLU, 因爲 mean and logvar 可正可負。
- 基於 reparameterization trick: $\mathbf{z} = \boldsymbol{\mu} + \boldsymbol{\sigma} \odot \boldsymbol{\epsilon} $ (20-dimension)
- VAE decoder 先是 FC network (fc3, 20->400) + ReLU
- 再串一個 FC network (fc4, 400->784=28x28) + sigmoid 保證介於 0~1 (to match mnist image grey level). 也就是 $\mathbf{f}$ = fc3+ReLU+fc4+sigmoid
- Forward path 包含 encode, reparameterize, decode.
1 |
|
VAE Loss function and optimizer
- 注意這裡VAE loss function 完全不用 label, i.e. 0, 1, …, 9. 可以說是 self-supervised learning.
- BCE 是 binary cross-entropy, 代表 reconstruction loss. 注意雖然稱爲 binary cross-entropy, label 可以是 0-1 的 value, 因爲 mnist 的 image 是 grey level 而非 binary value. 爲什麽是 reduction = sum 而非 mean?
- KLD 是 KL divergence, 是 regularization term. 在 Gaussian assumption 有 analytical form.
1 |
|
整合 training code
- Training dataset (60K) 由 train_loader 載入。Mini-batch size 可由 command line 指定, default = 128.
- model(data) 完成 forward, 傳回 reconstructed image, mu, logvar 用於 loss computation with batch_size=128. 就是每張 image 的 loss 纍積 128 張。
- 接著每個 mini-batch 計算 backward and use Adam optimizer to update weights. 不過爲了避免雜亂,只有 log_interval (default=10) 才 print 一次 log, default = 128x10 = 1280.
- 每個 epoch print average training loss (default 10 epoches).
1 |
|
結果
- 每一次 log 是 128x10=1280, 大於 2% of 60K dataset per epoch.
- Epoch 1 average loss 很大:164. 到了 Epoch 10 average loss: 106. 基本已經 saturated. 這個 loss 包含 BCE and KLD.
- Total loss: Epoch 1 ~ 164; Epoch 10 ~ 106.
- KLD loss: Epoch 1 ~ 14; Epoch 10 ~ 25.
- BCE loss: Epoch 1 ~ 150; Epoch 10 ~ 81.
- BCE loss 就是一般 autoencoder loss 隨著 epoch 增加變小,但 KLD loss 變大,同時 regularize BCE loss saturate.
1 |
|
下圖左上和左下對應 epoch 1 的 reconstructed images 和 random generated images. 下圖右上和右下對應 epoch 10 的 reconstructed images 和 random generated images. 都是 20-dimension.
Appendix A - Solution of Gaussian Distribution of $D_{K L}(q_\phi(\mathbf{z})|p_{\theta}(\mathbf{z}))$
\[\begin{aligned} \int q_{\boldsymbol{\theta}}(\mathbf{z}) \log p(\mathbf{z}) d \mathbf{z} &=\int \mathcal{N}\left(\mathbf{z} ; \boldsymbol{\mu}, \boldsymbol{\sigma}^{2}\right) \log \mathcal{N}(\mathbf{z} ; \mathbf{0}, \mathbf{I}) d \mathbf{z} \\ &=-\frac{J}{2} \log (2 \pi)-\frac{1}{2} \sum_{j=1}^{J}\left(\mu_{j}^{2}+\sigma_{j}^{2}\right) \end{aligned}\]And:
\[\begin{aligned} \int q_{\boldsymbol{\theta}}(\mathbf{z}) \log q_{\boldsymbol{\theta}}(\mathbf{z}) d \mathbf{z} &=\int \mathcal{N}\left(\mathbf{z} ; \boldsymbol{\mu}, \boldsymbol{\sigma}^{2}\right) \log \mathcal{N}\left(\mathbf{z} ; \boldsymbol{\mu}, \boldsymbol{\sigma}^{2}\right) d \mathbf{z} \\ &=-\frac{J}{2} \log (2 \pi)-\frac{1}{2} \sum_{j=1}^{J}\left(1+\log \sigma_{j}^{2}\right) \end{aligned}\]Therefore:
\(\begin{aligned} -D_{K L}\left(\left(q_{\phi}(\mathbf{z}) \| p_{\boldsymbol{\theta}}(\mathbf{z})\right)\right.&=\int q_{\boldsymbol{\theta}}(\mathbf{z})\left(\log p_{\boldsymbol{\theta}}(\mathbf{z})-\log q_{\theta}(\mathbf{z})\right) d \mathbf{z} \\ &=\frac{1}{2} \sum_{j=1}^{J}\left(1+\log \left(\left(\sigma_{j}\right)^{2}\right)-\left(\mu_{j}\right)^{2}-\left(\sigma_{j}\right)^{2}\right) \end{aligned}\) When using a recognition model $q_{\phi}(z|x)$ then $\mu$ and s.d. $\sigma$ are simply functions of $x$ and the variational parameters $\phi$, as exemplified in the text.
-
Reference: https://spaces.ac.cn/archives/5343 ↩
Reinforcement Learning
Markov Decision Process
-
States
-
(Transition) Model: transition matrix T(s, a, s’) = Pr(s’ \mid s, a)
-
Actions: up, down, left, right A(s)
-
Reward: R(s) or R(s, a) or R(s, a, s’) all math equivalent
- Markovian property: only present matters for the transition model.
- Stationary
Solution => Policy: $\pi(s) \to a$ and $\pi^*$ = up, up right, right, right
Why policy instead of a plan (trace)
- work everywhere
- robust against probabilistic model *
- Delayed reward
- Minor reward changes matter => reward is domain knowledge